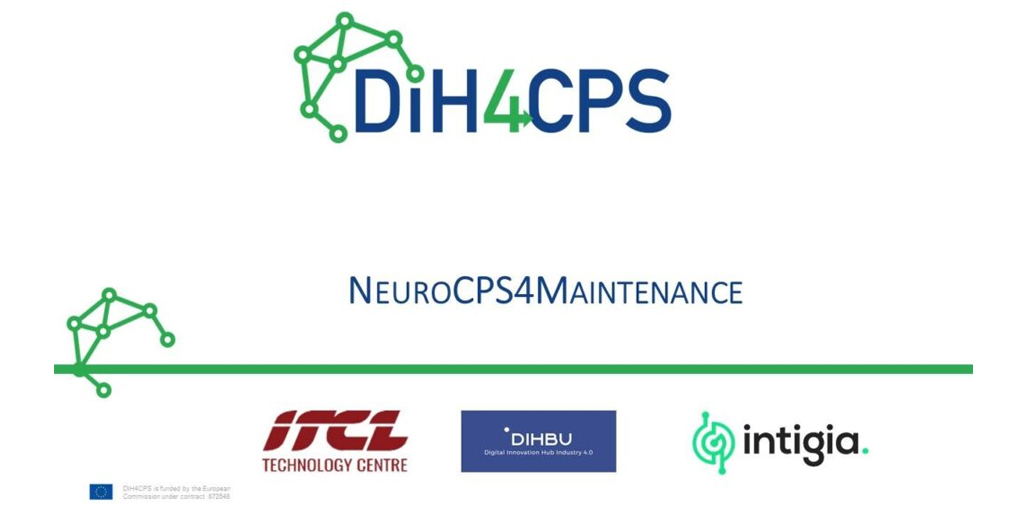
NeuroCPS4 Maintenance – Neuromorphic Anomaly Detector
NeuroCPS4Maintenance is a project that aims to develop and demonstrate a neuromorphic edge anomaly detector that is robust against conceptual drift, alerts to faults early and provides fast, real-time response for predictive maintenance applications in high-demand industrial scenarios (industrial press). This anomaly detector will be based on deep learning algorithms (LSTM) and implemented on system on chips (SoC).
Project Description:
The main objective of NeuroCPS4Maintenance is to develop and validate a new type of sensor, based on neuromorphic technology, capable of detecting anomalies, and alerting of failures in advance, and providing fast and safe real-time response, for predictive maintenance applications in high-demand industrial scenarios. This anomaly detector will be based on deep learning algorithms (LSTM) and will be implemented on system on chips (SoC).
The project will develop a neuromorphic anomaly detector and deploy and evaluate it in a relevant scenario.
Novel approaches will be used to develop this neuromorphic anomaly detector.
The development and demonstration of the neuromorphic processor will make extensive use of CPES technologies.
The LSTM-drift algorithm and hardware accelerators will be developed to implement it in real time and deploy the prototype in an industrial press (relevant environment), where its components can be validated.
Problem they aim to solve:
NeuroCPS4Maintenance aims to overcome the difficulties faced by SMEs in deploying predictive maintenance solutions due to lack of data sets and cybersecurity issues.
It will open the possibility of future applications of the system to other types of machines or robotic units, especially in advanced manufacturing processes, leading to applications with high market potential.
This consortium can be a first seed within DIH4CPS for the development of solutions based on neuromorphic edge processing for predictive maintenance replicable across Europe, and a first step for new use cases in robotics applied to other non-industrial sectors.
Project Resolution Objectives:
The common objective of the project is to solve these difficulties by generating new solutions that can be applied in a short period of time, through specific applications for each type of machine and maintenance problems.
The innovation capacity of this neuromorphic anomaly detector prototype will favor the enabling of new technical solutions in predictive maintenance in highly demanding industrial environments.
Project Duration:
2021 – 2022
Cooperation project with:
It is a project financed by ERDF funds through the DIH4CPS project financed by the European Commission (No. 872548).
Contact Person:
Javier Sedano, R&D Director – javier.sedano@itcl.es
Proyectos relacionados:
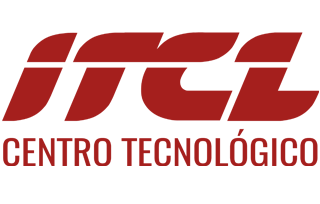