Soft Computing Solutions
ITCL provides several Soft Computing solutions focusing on the implementing of machine learning in hybrid systems, data analysis, feature selection, system modelling, and system classification and optimization. Furthermore, we also develop system processing bioinformatic solutions, clustering, and classification and modelling of dynamic series in gene expression profiling.
SOFT COMPUTING for dynamix series classification in gene expression profiling
Our won Soft Computing comprises several algorithms for co-expressed genes grouping in data analysis microarrays (MDA). Suitable for researchers trying to determine relevant genes and their co-expressed relations for large dynamic data sets so that an output feature can be optimised
Some of the included algorithms are:
- Shape Index (SC). Grouping dismissing the output of each sample.
- Output Shape Index (SOC). Grouping according to the gene correlation with the output.
- Dynamic Shape Index (DSC). Dynamic version of SC.
- Output Dynamic Shape Index (DSOC). Dynamic version of SOC.
- Relaxed Shape Index (RSC). SOC method enhancement.
The software includes fusion methods that combine in just one group every grouping created from each of the independently generated temporal series of microarray data. The most relevant clusters detection among the available ones is performed by using several measurements on the genes, such as Information Correlation Coefficient (ICC), Pearson Correlation Coefficient (PCC) and Shape Increase measures.
Machine variables optimisation from models
Self-designed Soft Computing model for the, optimisation of machine parameters in manufacturing processes including the development of models to assess the behaviour of the machine variables in the process and to determine the aptitude function to be optimised.
This process is carried out by obtaining the parametric objective function, or black box function, of the system. The machine parameters are then optimized by means of single-objective and multi-objective genetic algorithms, simulated annealing or ant colony algorithms.
An example of objective selection on a manufacturing process with three objectives is now displayed.
- Graph 3-D. The x axis represents an input, the Y axis another input to optimize and the Z axis the output.
- Graph 2-D. The x-axis represents an input and the Y-axis output.
- 3-D Graphics. Result of a multi-purpose search
Vehicle route optimisation system
Design of different hybrid route planning evolutionary algorithms for several vehicles, minimising the costs linked to transportation by: reducing the total trip duration, shortening the total distance, reducing waiting times, reducing the number of vehicles used, etc. While meeting a series of several restrains and requirements.
Main elements:
- Clients/delivery and pick up points
- Storage facilities
- Fleet (Vehicles capacity)
- Transportation network
- Function to be minimised
Modelling:
Modelling of the optimisation problems as different types of VPR (Vehicle Routing Problem):
- VRP with Pickup and Delivery (VRPPD): some clients are pickup points while some others are delivery points.
- VRP with Time Windows (VRPTW): clients must be served within specific Time Windows.
- Capacitated VRP (CVRP): vehicles have limited load capacity.
- Distance-Constrained VRP (DVRP): maximum allowed distance in a route.
- Multiple Depot VRP (MDVRP): multiple storage points.
Since VRP poses a complex combinatory optimisation problem (NP-hard) different heuristic techniques have been developed to solve the VRPs: Genetic Algorithms, Swarm Intelligence and Ant Colony Systems.
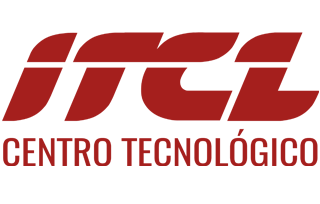